Why does that rain in the long-range forecast never seem to arrive? More often that not, if you look at your long-range forecast you’ll see some rain amounts. It’s not always the case, but usually if you look far enough into the future, rain is forecast somewhere. But why is it that this rain often never actually arrives? In this blog post, we take a closer look at this commonly asked question. To learn more about our forecasts, click the button below:
To tackle this rain question, we first need to start by looking at how weather forecasts are made. In general, forecasts fall into one of two categories: either fully automated, meaning made entirely by computers, or meteorologist-edited. In the meteorologist-edited forecast, computer forecasts are tweaked by meteorologists to try and improve their accuracy. However, there are very few large weather forecasting agencies that actually have their meteorologists modify the long-range forecasts – why, you might ask? Well, this has to do with the “forecasting continuum”. It is generally accepted that meteorologists add the most value to the forecast in the short-term and less value in the long-term. Due to the complexity of weather, long-range forecasts are generally better handled by computer models that can consider the countless factors that affect these forecasts. Therefore, we must really ask why computer models almost always produce rain in the long-range.
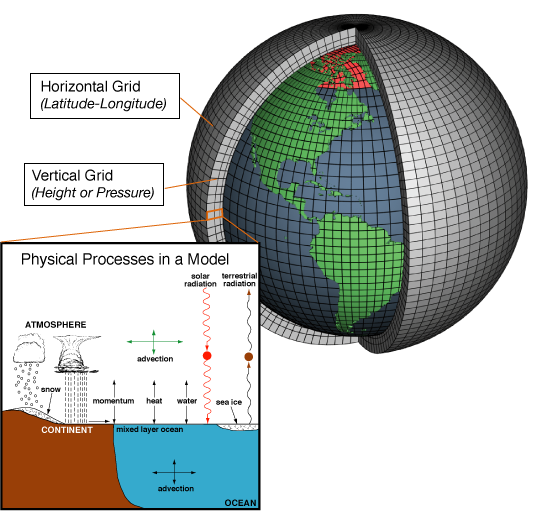
When it comes to weather models, there are two main categories: deterministic or ensemble. In simple terms, a deterministic model is a single weather model forecast. On the other hand, ensemble models are when many weather models are run at the same time, to produce a wider range of outcomes. Ensemble models usually include as few as 20 or as many as 50 different members, or forecasts. All of these different model simulations are averaged to produce the forecast you see.
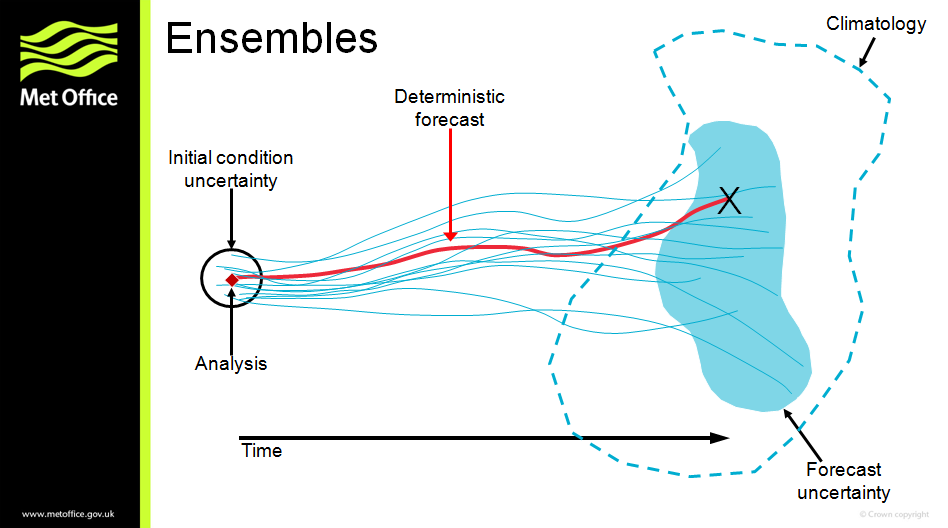
Some weather agencies may use deterministic weather models for the long-range forecast. However, this is usually considered to be a bad practice, and ensembles are strongly favoured when it comes to producing long-range forecasts – therefore, we will assume the forecast you are seeing is ensemble-based. Take the following table as an example:
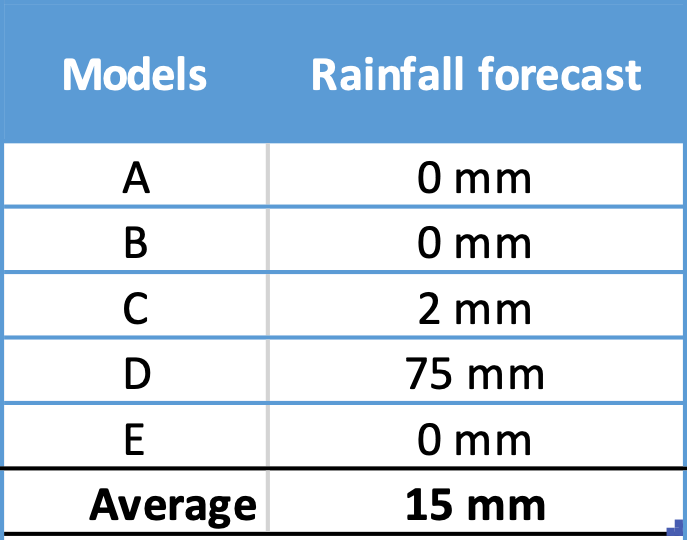
In the example above, there are five model forecasts shown, labelled A to E. As mentioned earlier, most ensembles are an average of more than 5 forecasts, but we’ll just use five here to keep it simple. In this case, 3 of the 5 models are predicting no rain, one model is predicting 2 mm, and another model is predicting 75 mm. If you average all 5, the forecast is 15 mm. You may be wondering, why wouldn’t we just give a forecast of 0 mm, since all the models but one show almost no rain? This is a valid question and in fact a meteorologist might indeed determine a forecast of 0 mm is most valid. However, as also mentioned before, these forecasts are entirely automated. Furthermore, it starts to defeat the purpose of an ensemble if you start tossing out some of its forecasts. An ensemble is supposed to predict a range of outcomes and therefore we need to assume models A-E are all equally valid. In fact, the power of an ensemble is the ability to assign probabilities!
With a deterministic model there is one forecast and therefore only one predicted outcome. With an ensemble, there are many predicted outcomes, so we can assign probabilities to those different outcomes. In this case, four of five models predict 2 mm or less, therefore we could say the probability of less than 2 mm is 80%*. Conversely, the probability of 75 mm is 20%*. The ability to give objective probabilities is one of the most powerful features of ensembles! The image below shows a map with probabilities of receiving at least 20 mm of rain over a 24 hour period:
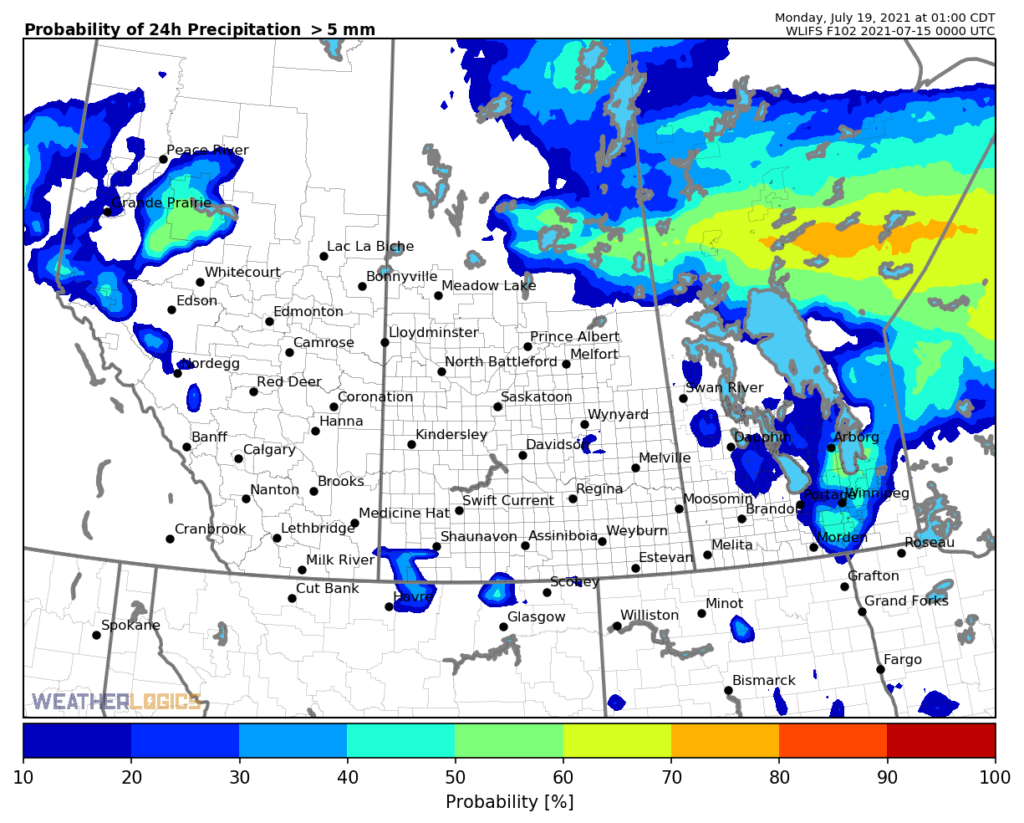
In the previous example you saw a good example of how ensemble are used to make forecasts, but let’s get back to the original question – why is there often rain in the long range? As you probably already know, the longer the forecast is, the more errors increase. A forecast 2 days from now is far more accurate than a forecast 10 days from now. As a result, a forecast 10 days from now will have a much larger spread, or range of forecasts. Ten models might say 0 mm, five might say 25 mm, another 5 might say 50 mm, and so on. Because the long-range forecast has a larger spread in outcomes, inevitably some models will be producing large rain amounts. Therefore, when all these outcomes are averaged, even a few large amounts in the long-range, say three models predicting 75 mm, while the rest produce almost nothing, will skew the average higher. As a result, you see those teaser amounts in the forecast.
In summary, why does the long-range forecast always have rain? Because in the long-range, errors increase and there will almost always be a wide range of different model forecasts. Therefore, at least some models will almost always be producing rain, sometimes large amounts, whereas in the short-term the range is smaller and you won’t often get some models producing large amounts if others aren’t. The averaging technique that is used to present the forecast will therefore give rainfall amounts in the long range, which may disappear as the forecast converges later on.
At Weatherlogics, we use advanced statistical techniques to help eliminate these large “teaser” amounts in the long range, unless there is good reason for them to be there. Our meteorologists also provide expert written outlooks, which help describe if the long-range pattern favours heavy rain. To learn more, visit our agriculture page!
Footnotes
*In reality more complicated probability distributions are used in these calculations, but we are simplifying the calculation for this example.